How I teach the meaning of (statistical) p-values using variation theory
- Christian Moore Anderson
- Jan 23, 2024
- 4 min read
Updated: Nov 28, 2024
A principle of variation theory is identifying the aspect you want students to discern and then varying it. When you vary it, keep all others invariant so that students notice what you want them to.
To understand p-values, you must discern a critical aspect: how confident you can interpret data. You must see how the relationship between the means and their data spread can vary, and how this affects your confidence in interpretation. This post is about getting students to discern the meaning of p-values. Here's how I do it with just diagrams and dialogue.
I begin by drawing a representation of sampling. This is a key concept but students are often familiar with it due to the exams they sit.
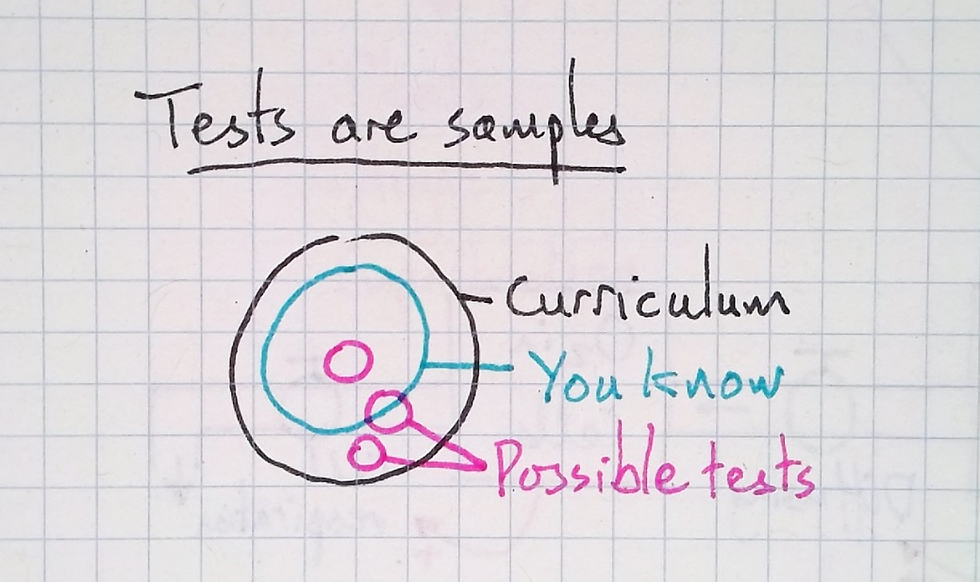
The largest circle represents all the information in a curriculum. The medium circle represents how much of this a student knows. The three smaller circles represent the knowledge that may be sampled on any individual test.
If you’re lucky, a test samples the part of the curriculum you know. If you’re unlucky, it’ll test everything you don’t know. Most of the time it’s somewhere in between. I ask students if it’s better to have a larger or smaller exam as a sample and they agree that a larger sample is less risky. I point out that it’s also more reliable as a measure of what they know.
Next, I draw a bar chart representing two classes—studying the same course with the same teacher—and their test results. Both classes score 50% on average and I ask the students if the classes are identical.
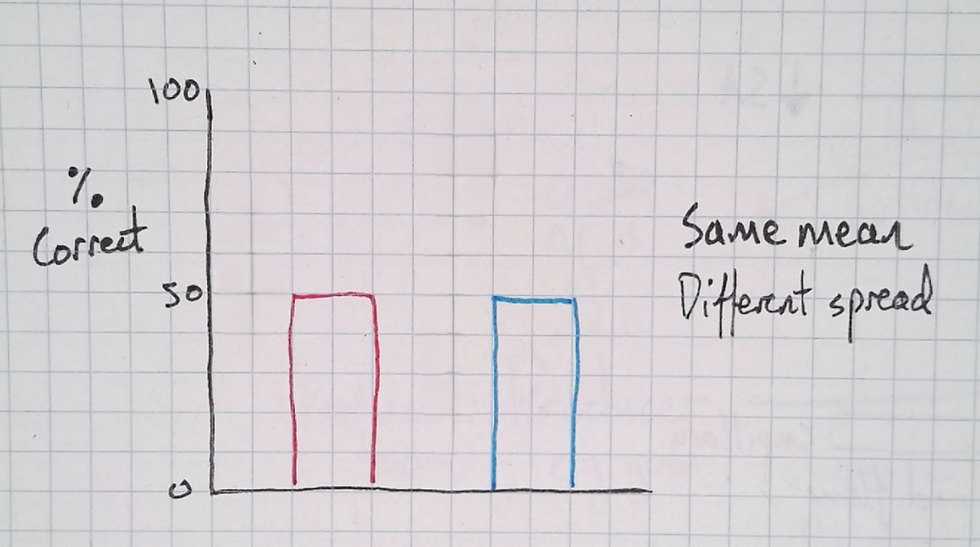
While some students suggest that they are, some students sometimes mention that one class might have more higher, and lower scores than the other. I add this to the bar chart using dots to represent individual scores.
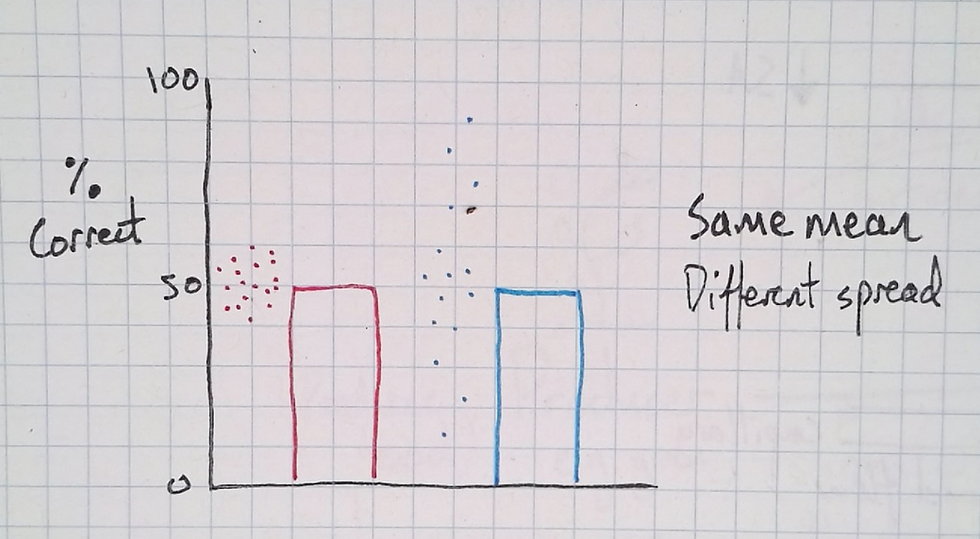
The scores of one class spread little from the mean, whereas the other's spread further from their mean. This step has varied one thing. It has kept invariant the curriculum, the exam, and each class’ mean result; only the spread of results has varied. This, then, has brought it to the attention of the students.
I then draw a bar chart with identical axes, and I say to students that, in the same course, two other classes got these results:
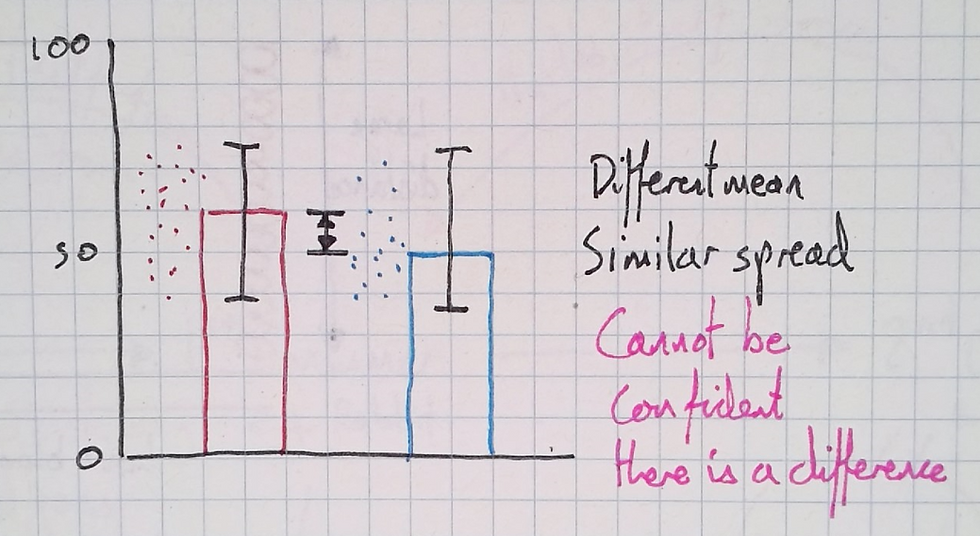
Now the means are different and I draw dots to show a similar spread of results. As the means vary but the spread doesn't (much), it is brought to the students' attention (forgive the range bars here instead of standard deviation bars). I ask the students if the classes are different—does one class know more than the other?
To help them here, I ask if they think one class would always come out better, on average, if I gave them five more tests to complete.
Crucially I ask them how confident they would be making a prediction. This is key to understanding p-values, as "p" refers to probability. The students typically agree that they couldn’t be so confident that they’d keep getting the same difference between the classes in other tests. Therefore, the classes could be similar in overall knowledge.
I then draw another bar chart with identical axes. This time, however, both the mean and the spread are different. The means represent 80% correct for one class and just 30% for the other. And each class' individual results don't overlap; far from it.

I then repeat the question to the student; how confident are they that the two classes are different in overall knowledge? Again, I ask what would happen if I gave them five more tests. The students typically express that they would be very confident that they could predict the result.
Next, I give students a heuristic for interpreting graphs with error bars. I draw the error bar examples one by one and ask students if they would assume that it was a real difference. The a rough rule of thumb I give them is to only assume a difference if the error bars do not overlap at all, but to remember that this is an assumption only. In fact, some would give a stricter rule.
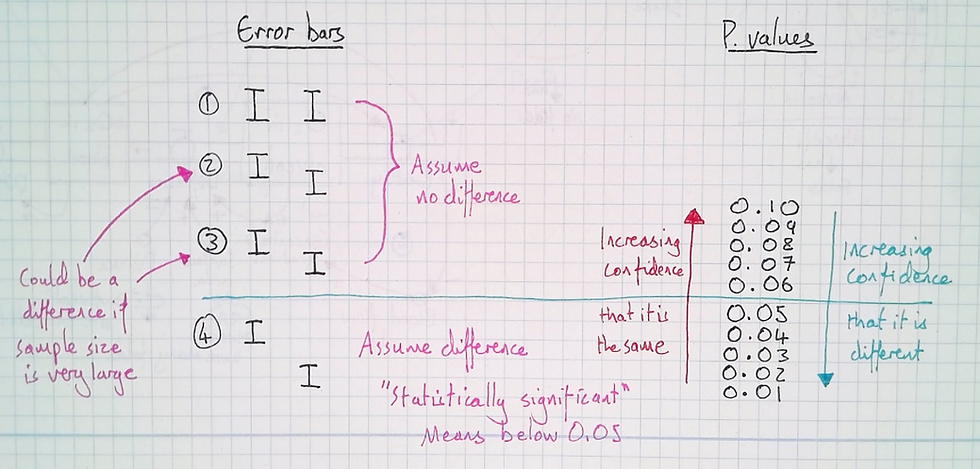
I also give them the exception that error bars may overlap and still represent a difference when the sample size is very large. With this, they should seek better verification of how confident they can feel. Here then, I introduce the idea of the p-value and tell them that many different statistical tests will give a p-value; a number that indicates a statistical level of confidence. Not a magnitude of difference, but a level of confidence you can assume that there is a difference (large or small).
I finish the lesson by discussing the typically acceptable p-values—below 0.05—the standard for being confident enough to assume a difference. Higher than this number you should be more inclined to assume similarity. In biology, as data is messier, a p-value of 0.05 is more acceptable than in physics in which systems being observed are simpler and can be controlled with precision.
Finally, I discuss the emergent problems when scientific journals prefer to publish papers with p-values below 0.05, which incentivises p-hacking and the loss of information about experiments that reveal similarities rather than differences. This is teaching with diagrams and dialogue; do you want to co-construct meaning without lecturing, slide decks, or leaving students to discover for themselves? Learn how and why in my books. Download the first chapters of each book here.